Data as a Service
Data as a Service (DaaS) is our term for serving Data as a utility in the organization: reliable, quality Data with built-in governance, available on demand, and easy to use.
This utility-based model provides Enterprise Data Access for applications, AI/Data science needs, business users, and external parties via seamless microservices API-based access to analytics and Data extracts with proper access management and monitoring.
The Data warehousing operations are managed by a dedicated team whilst shielding users from the complexity of its implementation.
Getting Data foundation right for positive business impact
[noun]
The provisioning, distributing and analyzing of Data in organization whereby Data management, governance and security is inherent to each Data element at Group or Business Area Layers.
Built-in Data Governance
Improves Data Quality
Data Science at Scale
Reduces Cost
Real Time Processing
.
Built-in Data Governance
.
Improves Data Quality
.
Data Science at Scale
.
Reduces Cost
.
Real Time Processing
Data as a Service Pillars
Open
Architecture
Future ready Architecture API driven approach for ingestion and publishing of analytics and Data extracts.
Self
Service
Data and analytics on-demand logical, federated view of Data business autonomy.
Governance
is the key
Data standardized across a single platform defined policies on use of Data throughout its lifestyle.
Driving
Data Culture
Data culture and Data success are intertwined and interdependent.
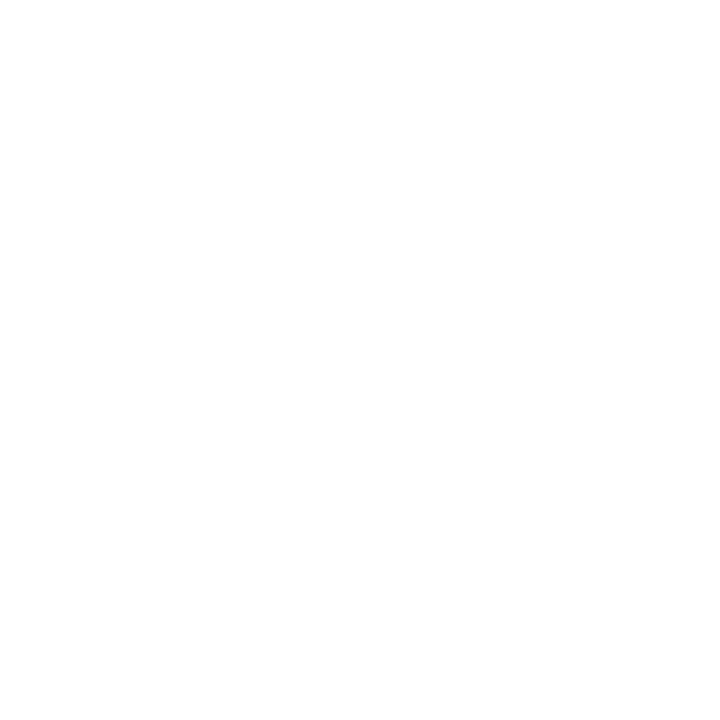
Open Architecture
Future ready Architecture API driven approach for ingestion and publishing of analytics and data extracts
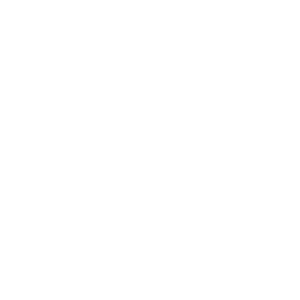
Self Service
Data and analytics on-demand logical, federated view of data business autonomy
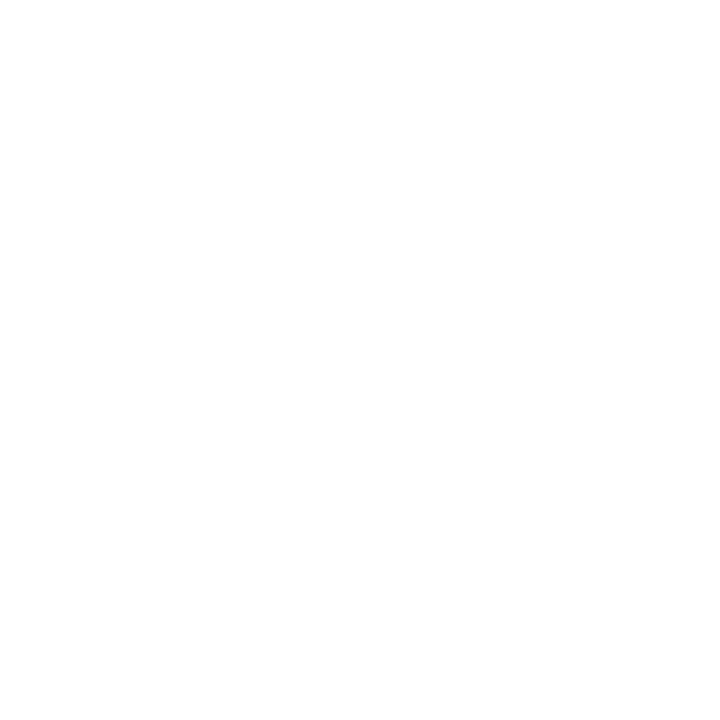
Governance is the key
Data standardized across a single platform defined policies on use of data throughout its lifestyle
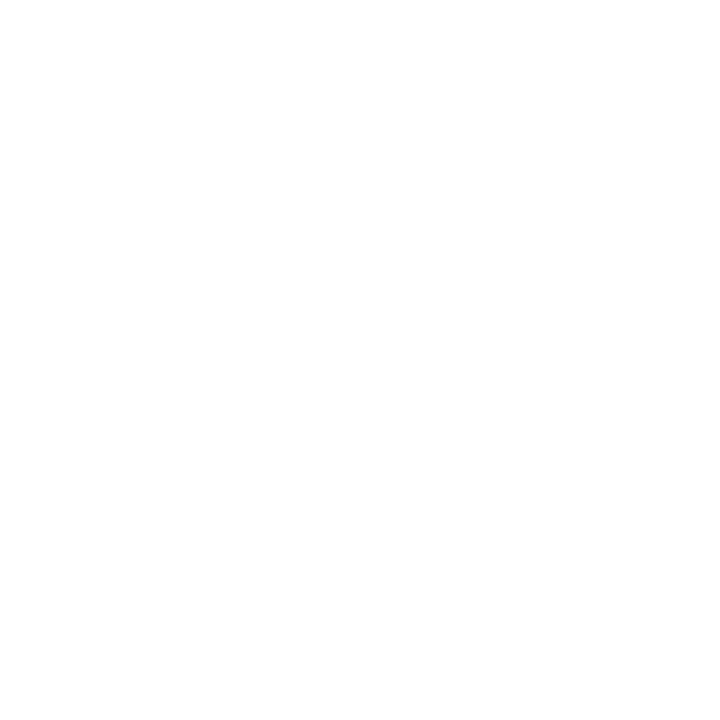
Driving Data Culture
Data culture and Data success are intertwined and interdependent
Open Insights’ approach to build DaaS
Rapid assessment of current and target state
Stand-up partial DaaS supporting first high-value use case
Open Insights’ DaaS Principles
-
1
Open Architecture & Data APIs
-
Utilizes open source technologies and open storage formats to leverage ongoing innovation and to reduce TCO
-
Allows for integration with open source Data sharing and proprietary tools
-
-
2
Data Acquisition & Access
-
Supports ingestion and storage of Data from the most granular (structured & unstructured) to the most aggregated
-
Able to ingest Data from existing and future sources in agile model examples (as a side note/sidebar): payments Data, Point of Sale, product sales, transaction Data, subscriber details, and loan Data
-
-
3
Continuous/Real-Time Metrics/KPIs and Decisions
-
Engine updates metrics and calculates decisioning variables based on streaming (real-time events) Data combined with historical events
-
Executes analytical models (predictive, rule based) in real-time provides a real-time view of the business and performance indicators via BI and operational dashboards
-
-
4
Powering AI, Machine Learning and Business Intelligence
-
Enables analytic models to automatically calibrate and tune themselves based on decisions generated from the underlying engine
-
Business and operational users can tag decisions and alerts as false or true positives, as well as identify false negatives, to improve the self-learning matching and decision process
-
-
5
Built-in Data Governance
-
Improved Data quality controls and transparent Data lineage help ensure internal and regulatory compliance
-
Continuous logging and monitoring assure robustness and auditability of the Data pipeline
-
Information security is governed and applied for all Data
-