Rapid Build & Implementation
Through many deployments, we have evolved reference architecture for the DaaS. However, building an entire DaaS at once is a bad idea. We de-risk our build approach towards our reference architecture by dividing it into small parts. Each part is designed to deliver high-value use cases within a 12-week sprint. By building in small pieces towards reference architecture, we avoid creating messes and ensure relevance to the clients’ business needs.
Build in parts
to a reference
architecture
Each part delivers business value in weeks
Agile approach in
collaboration with
your organization
DaaS iteratively
completed via
sequential use cases
Is Data an asset or a liability?
Without a clear action and correct management of Data, organizations are at risk of “hoarding” Data and letting it sit idle, turning it into a cost center and risk driver: externally hackable or internally leakable. We securely add value to the Data, enabling organizations to deliver their services intelligently to a highly digitalized world.
How do you build Data as an asset?
Data as an asset becomes your organization’s “brain” by continuously capturing interactions and coding them into actionable knowledge. It makes Data more than just numbers in your reports.
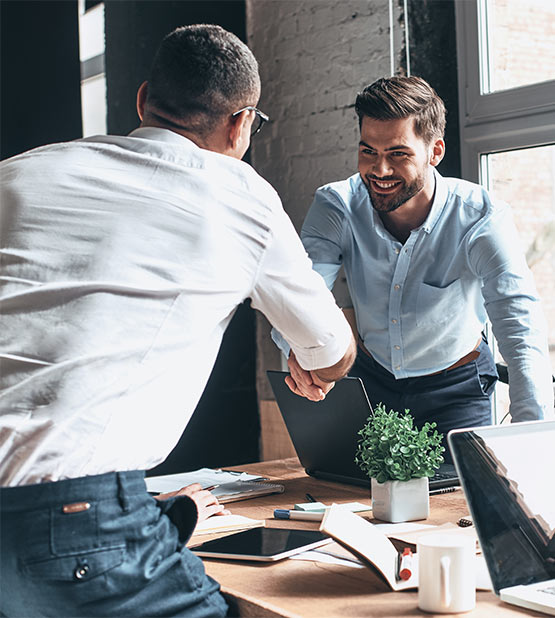
Predictive Analytics
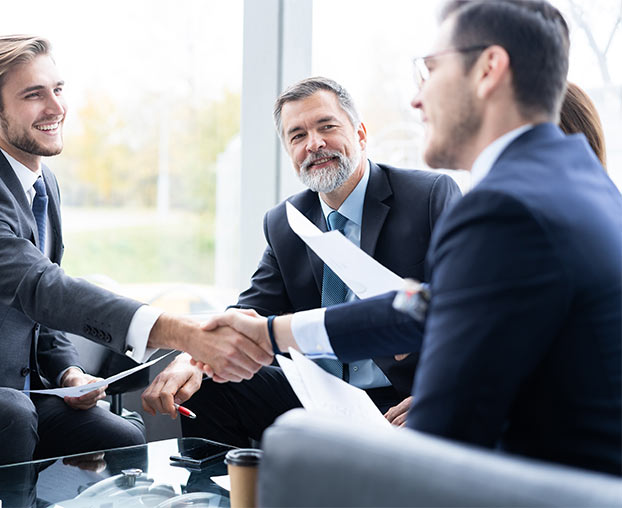
Predictive Analytics
Use advanced algorithms to predict various customer behaviors at micro and macro levels
Strengthen marketing, ad targeting and attribution models
BigData Implementations
Bring up on-prem or cloud-agnostic BigData infrastructure
Migrations from expensive Data warehouse appliances to affordable performant commodity or cloud-native hardware with open source software Data platforms
Iterate
Productionize
Iterate
< 2 weeks
Hothouse
Articulation of business needs, benefits and success criteria
Roles and responsibility
Roadmap and planning
Governance setup
Metadata management approach
4 - 6 weeks
Design and prove the solution
Architecture and design
Infrastructure Setup
Data analysis
Security setup
Metadata tool
Built in Data quality
Data science and analytics
8 - 10 weeks
Iterative framework and build
DevOps and CI established
Reusable framework components build
Automated testing
Feedback from business
Iterate and enhance framework
12 - 14 weeks
High value use cases
Use case delivery/ Roll out
Launch in multiple departments / groups / countries in waves
Iterate 
Self sufficiency
Onboarding of new use cases / Country with minimal technology effort